
How to Protect Data and Devices at Scale
Readying Your Data and Infrastructure for AI
Navigate future complexities and leverage the transformative power of AI for data and device protection through strategic visualization and development of organizational goals.Read this article for key insights that include:

How to Protect Data and Devices at Scale
Readying Your Data and Infrastructure for AI
Navigate future complexities and leverage the transformative power of AI for data and device protection through strategic visualization and development of organizational goals.Read this article for key insights that include:
Starting with a Vision
According to Microsoft, three-quarters of knowledge workers (75%) use AI, with 46% adopting it in just the last few months. Rarely does a new technology take off so rapidly. At the same time, according to Cisco, 55% of business and IT leaders say their technology infrastructure is not prepared to handle AI. That’s because new AI-powered tools require new hardware, data, and skillsets. They also require addressing AI’s unique security challenges.
However, thoughtful preparation and best practices for approaching infrastructure upgrades and data management can go a long way toward helping organizations prepare for AI.
It starts with a vision.
Starting with a Vision
According to Microsoft, three-quarters of knowledge workers (75%) use AI, with 46% adopting it in just the last few months. Rarely does a new technology take off so rapidly. At the same time, according to Cisco, 55% of business and IT leaders say their technology infrastructure is not prepared to handle AI. That’s because new AI-powered tools require new hardware, data, and skillsets. They also require addressing AI’s unique security challenges.
However, thoughtful preparation and best practices for approaching infrastructure upgrades and data management can go a long way toward helping organizations prepare for AI.
It starts with a vision.
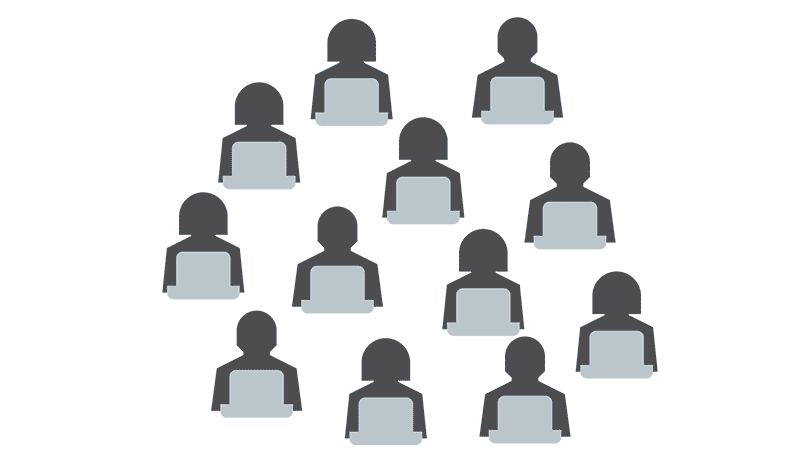
Three-quarters
Change Happens. EXPERTISE WINS.™
Power up your team with the latest modern infrastructure solutions to drive greater productivity, collaboration, and security.Talk to an Expert
Strained Infrastructure and Siloed Data
Data is critical for effective AI implementation, and it must be properly managed, yet most organizations are unprepared. Eight out of ten (81%) respondents to the Cisco survey report that their data is locked up in silos, stuck in individual applications, and hardware that can’t talk to each other. Compounding the problem is unstructured data from sources like emails and text documents mixed in with structured, more easily machine-readable data in databases. Data migrated to clouds without proper governance is another complicating factor. All of which can significantly hinder the groundwork required for AI adoption.
In fact, according to Gartner, only 4% of IT leaders characterize their data as AI-ready. But Gartner also offers a roadmap for moving forward. Step one: get clear-eyed about what organizational leaders want to accomplish with AI. A well-defined AI vision encourages organization-wide adoption, helps secure funding for valuable use cases, and drives further innovation.
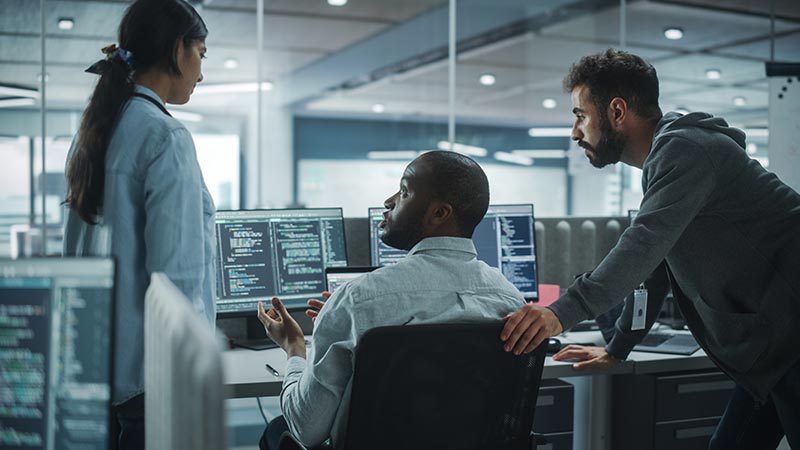
With a vision articulated, an organization can prepare the groundwork for AI.
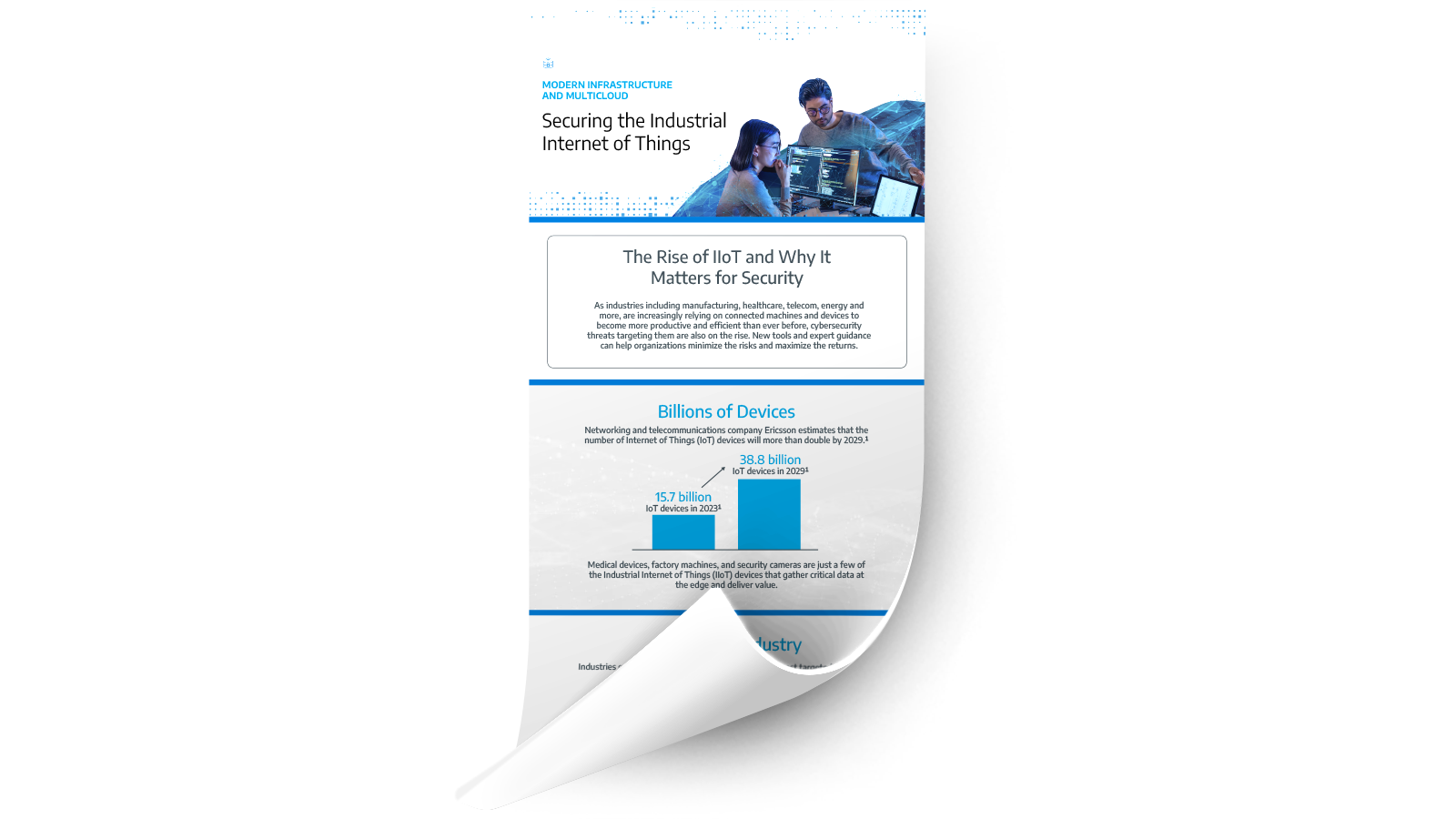
Securing the Industrial Internet of Things
Check out our infographic for facts and figures on the rise of IIoT and why it matters for security.
A Practical Guide to Zero Trust Implementation in Multicloud Environments
Read our eBook for a five-step plan that will help accelerate your journey to Zero Trust.Laying the Groundwork for AI
Gartner suggests using a scoring system to rate and rank potential AI projects, evaluating both technical feasibility and anticipated business value for proposed use cases.
A scoring system can take into account technical factors such as access to labeled data, IT architecture, and the availability of skilled personnel. Effective business value factors include alignment with the organization’s mission and values, sponsor support, and measurable KPIs. Proposed projects scoring high in both feasibility and value represent areas of opportunity.
As for where to look for potential use cases, Gartner categorizes AI use cases into two broad categories: everyday AI and game-changing AI.
Everyday AI seeks to boost productivity and efficiency for existing processes. Functions ripe for AI-powered improvement might include back-office administrative tasks such as document processing. Customer-facing tasks might include writing support for sales and marketing staff as well as some customer service functions.
Game-changing AI aims to reinvent core capabilities and create new products or services. AI in this area has the potential to accelerate research and development, optimize supply chains, and reinvent internal operations. Customers might also benefit from new products and services made possible by AI.
Deciding what use cases to pursue depends on an organization’s overall tolerance for risk, timelines for achieving AI goals, and the organization’s industry and competitive landscape—among other factors.
Regardless of the circumstances, just about every organization in every industry stands to benefit from AI.
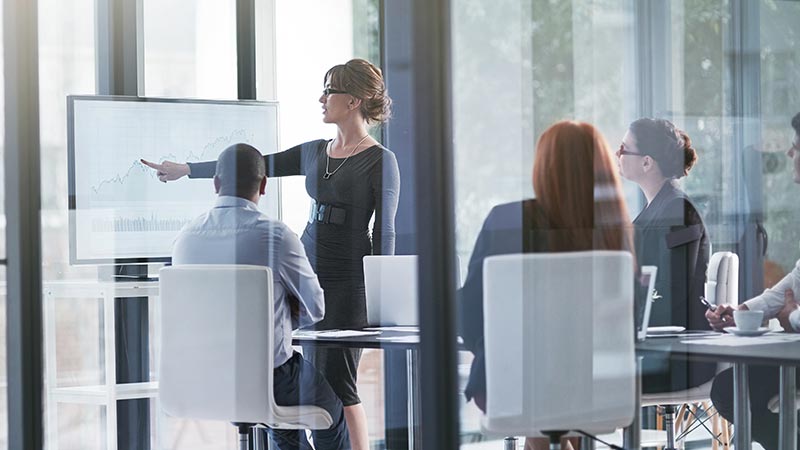
According to a recent survey by IDC:
- 36% of healthcare organizations are realizing three times their return on AI investment.
- The retail and consumer packaged goods sectors are doing even better, with 40% of survey respondents reporting the same results.
- Nearly a third of respondents in government and education report gains of four times their return on AI investments.
- And nearly half of respondents in manufacturing (45%) are getting back three times their return on investment (ROI).
ROI may be directly measurable or indirectly hidden in other components of the business such as operational efficiences. The opportunities are there if organizations empowered with a clear vision and aligned goals know where to look for them.
Assessing Critical Infrastructure Elements
- Hardware: Ensuring sufficient compute power through upgraded devices that can handle the demands of large AI models. This may require more powerful servers and storage arrays—including access to GPUs.
- Network: Investing in network infrastructure with the bandwidth needed to process large amounts of data and return results quickly to users in a secure fashion—especially in edge computing use cases. Notable near-term development in 5G will be important to watch and consider.
- Data: Putting data hygiene, governance, and management practices in place before using data for AI applications. This preparation work will benefit from robust data management tools capable of data-at-rest and data-in-transit analysis and alignment with existing security tools. It also requires a team effort between IT and business units across the organization.
- Security: Strengthening security measures—such as Zero Trust protocols—can help to safeguard the increasingly valuable data used for AI as well as any AI systems and models the organization might develop. To learn more about the advantages of Zero Trust continue to our eBook.
- Cloud: Leveraging cloud technologies, including native AI tools, for efficiently realizing compute and data storage at scale—along with layers of security, both built-in and extendable. Hyperscalers and advanced data center providers can rapidly accelerate the timeline to implement use cases.
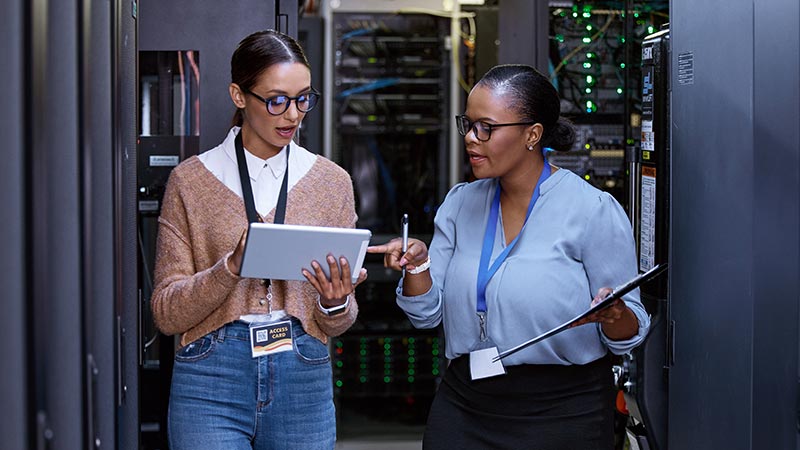
Next Steps
From there, organizations can make any necessary hardware upgrades to support the increased computing power and network bandwidth required by AI models. Knowing in advance what AI applications and workloads to prioritize helps avoid over-investing in on-premises infrastructure and over-provisioning cloud resources.
The old IT adage “garbage in, garbage out” (GIGO) is especially true of AI applications. Ensuring that data is clean, well-organized, and accessible is critical for achieving organizational goals for AI workloads. Look to modern data federation and governance solutions to enable effective, safe, and secure AI.
Finally, consider that you may not have the people resources you need to accomplish your goals for AI in the timeframes you envision.
You wouldn’t be alone. In a recent IDC survey, most organizations (52%) cited a lack of skilled workers as a primary factor hindering AI adoption. Therefore, partnerships with hyperscalers and advanced data center providers may be necessary.
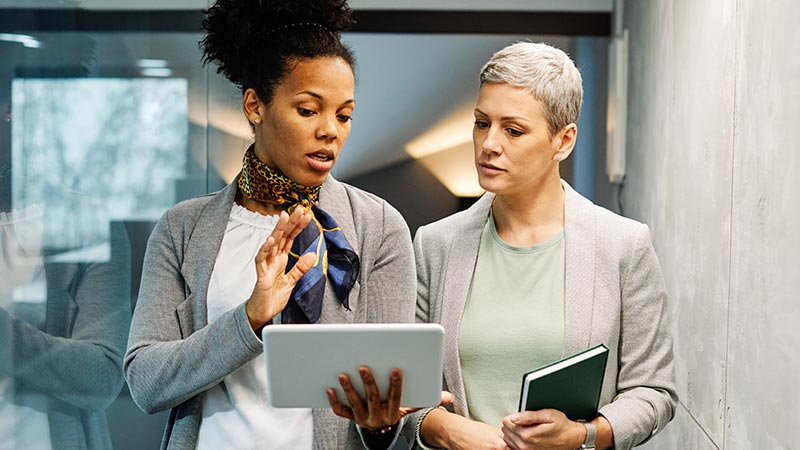
Protect Data and Devices at Scale with Modern Infrastructure and AI solutions.
Talk to our experts today!
Learn MoreConnection Community
Securing IoT Devices, Tools, and...
Manufacturing has made the digital transformation. The good news is that digital factories are more efficient and have better production... Read More
8 Steps for Securely Readying Your Data and...
AI has almost reached the point of becoming as ubiquitous to an organization’s IT system as cloud computing. In fact,... Read More
Federal Health IT Strategic Plan and Modern...
This image was generated by AI. In my first three Connected Blog posts in this series, The Federal Health IT... Read More
Top 8 Benefits of Backup and Recovery as a...
As businesses navigate an increasingly interconnected and data-driven landscape, the stakes for data loss or unauthorized access have never been... Read More
Bulk Up Security with SIEM and XDR
Ransomware attacks have increased significantly due to the shifting remote and hybrid workforce. Your organization’s IT structure now spans across... Read More